Installer des panneaux solaires sur les camions frigorifiques, dématérialiser les processus dans les entrepôts, utiliser des données précises en temps réel pour gérer le coût environnemental des livraisons par produit et par itinéraire… Ces initiatives, et bien d’autres, sont au centre des préoccupations des leaders de la Supply Chain, qui s’efforcent de rendre cette dernière plus durable et d’alléger le fardeau pour la planète.
Si ces actions figurent en bonne place dans l’agenda logistique, c’est que la durabilité est un levier important. Selon le Forum international des transports, les émissions de dioxyde de carbone devraient afficher une hausse de 16 % d’ici 2050, à la suite de l’augmentation du transport de marchandises – et ce, même si les pays s’engagent à les freiner. Si l’on n’intervient pas, les villes du monde entier accueilleront des millions de véhicules de livraison de fret et de colis en plus au cours de la prochaine décennie. Résultat : davantage d’embouteillages et une augmentation équivalente des émissions de gaz à effet de serre, comme le signale le Forum économique mondial.
La conclusion qui s’impose : il est primordial de trouver comment rendre la logistique et les livraisons plus durables du point de vue environnemental.
« Les entreprises s’intéressent de plus en plus à la durabilité des opérations logistiques », remarque Josué Velázquez Martínez, directeur du laboratoire des Supply Chains durables du Centre de transport et de logistique du MIT. « Elles font face à des pressions croissantes de la part du législateur et des consommateurs, et s’efforcent vraiment d’améliorer tous les aspects de la Supply Chain ».
Ces mêmes entreprises pourraient se trouver contraintes de prendre davantage de mesures. Si l’on se réfère aux règles en vigueur, nombre d’entre elles n’indiquent pas suffisamment les émissions générées par leur Supply Chain dans leurs rapports de durabilité, voire les omettent totalement, indique le New York Times. Or, il est possible qu’elles y soient bientôt obligées, car les autorités de régulation ont aujourd’hui la logistique dans le collimateur. Aux États-Unis, le législateur envisage de demander aux entreprises publiques de déclarer leurs émissions tout au long de leur Supply Chain. L’UE a également élaboré une proposition de directive qui renforce les règles de communication d’informations en matière de durabilité.
Les entreprises s’intéressent de plus en plus à la durabilité des opérations logistiques. Elles font face à des pressions croissantes de la part du législateur et des consommateurs.
Josué Velázquez Martínez, Centre de transport et de logistique du MIT
Les attentes des consommateurs, les réglementations et la demande du marché poussent les dirigeants d’entreprises à agir davantage sur le terrain de la durabilité. Tout naturellement, c’est au niveau des systèmes de logistique et de distribution que sont mises en place de nouvelles normes, qui contribueront largement à créer un avantage concurrentiel. En effet, les entreprises qui économisent sur la livraison en utilisant moins d’énergie et de ressources bénéficient d’un meilleur retour sur investissement (sur leur parc de camions, par exemple) et imaginent des modes de livraison plus efficaces. À cela s’ajoute, du côté des consommateurs, une conscience accrue du coût environnemental des livraisons et une volonté de choisir des options moins rapides pour préserver la planète.
Mais pour voir l’avènement d’une logistique durable, nous devons optimiser nos itinéraires de transport, construire des entrepôts plus efficaces et utiliser plus souvent et plus efficacement les données. Autant de tâches qui n’ont rien de simple. Avant de pouvoir réellement mesurer les progrès en matière de développement durable et agir sur l’empreinte carbone des produits, il y a de nombreuses étapes à mettre en œuvre.

Pas de temps à perdre: Découvrez pourquoi les entreprises doivent faire coïncider leurs ambitions et leurs actes en matière de durabilité.
Lire les actualités
D’après le rapport « Le paradoxe de la Supply Chain durable » établi par SAP et Oxford Economics, les cadres dirigeants ne connaissent pas encore suffisamment leur Supply Chain et éprouvent des difficultés à la rendre plus durable. Ils en sont encore à fixer des objectifs, et non à les mettre en application. Près de la moitié des personnes interrogées jugent que la complexité et le coût sont les deux principaux obstacles dans cette démarche de durabilité.
Mais comme l’explique Shimon Gowda, responsable de configuration de la Supply Chain chez Chainalytics, une pression croissante incite les entreprises à gagner en visibilité sur leur Supply Chain globale et à atteindre la neutralité carbone. « De plus en plus d’entreprises s’efforcent de mesurer l’empreinte carbone de leur réseau actuel », ajoute-t-il. « Les leaders du secteur mettent tout en œuvre pour élaborer une mesure précise et exacte, qui servirait de point de référence auquel comparer une situation à l’instant t. »
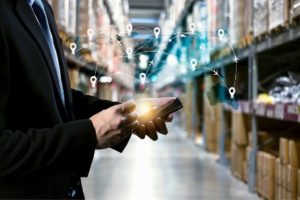
Pourquoi la logistique constitue un objectif de durabilité majeur
La logistique est une mission complexe. Elle consiste à gérer l’acheminement des matières premières, des produits intermédiaires et des emballages à travers le monde en direction des sites de production, mais aussi la distribution des produits depuis leur lieu de fabrication jusqu’au consommateur. Or, le transport fait partie des domaines dans lesquels l’objectif « zéro émissions » est un enjeu important. Il s’agit notamment de réduire les distances parcourues et d’utiliser plus efficacement les différentes options. Il n’est pas rare, par exemple, que des camions reviennent à vide une fois leur livraison effectuée.
Prenons l’exemple des transports frigorifiques : nous utilisons des camions réfrigérés pour transporter les produits pharmaceutiques, les fruits et légumes, les produits laitiers, la viande et les boissons. Cela nécessite de maintenir la chaîne du froid à l’intérieur de la remorque – ce qui consomme beaucoup d’énergie, traditionnellement de l’essence.
Certaines entreprises contribuent à rendre le transport frigorifique par camion plus durable en alimentant les systèmes de réfrigération à partir de sources d’énergie alternatives. eNow, par exemple, installe des panneaux solaires sur le toit des remorques. Quant à Coldtainer, il fabrique des boîtes de stockage pour la chaîne du froid.
Certaines entreprises s’efforcent de rendre le transport frigorifique par camion plus durable en alimentant les systèmes de réfrigération à partir de sources d’énergie alternatives.
D’après le média NPR, des entreprises bien établies telles que FedEx et DHL investissent dans des matériels et des équipements tels que des véhicules électriques. Mais M. Martínez en est convaincu : se concentrer uniquement sur de nouveaux véhicules est le meilleur moyen de manquer d’importantes opportunités.
« Je constate qu’on s’intéresse beaucoup aux équipements, ce qui est vraiment une bonne chose pour le long terme », explique-t-il. « Mais en attendant, il y a de nombreuses opportunités auxquelles les entreprises ne font pas vraiment attention à ce stade. »
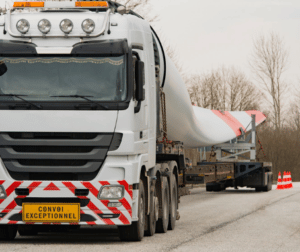
Lorsque votre kilométrage varie, choisissez le bon véhicule pour chaque itinéraire de livraison
Ces opportunités, qu’étudient notamment M. Martínez et bien d’autres personnes, ce sont notamment des systèmes de planification des transports qui optimisent les itinéraires, réduisent le kilométrage et les émissions, et analysent les effets de la topographie sur les performances des camions.
Renouveler sa flotte n’est pas, en soi, un mauvais choix. Mais un camion neuf n’est pas nécessairement la meilleure option, écologiquement parlant. Les recherches du laboratoire d’étude de durabilité des Supply Chains ont permis d’établir que le kilométrage, tel qu’il est mentionné sur les sites Internet des constructeurs automobiles, est divisé par deux environ en conditions de livraison réelles. L’écart tient probablement au fait que les tests des camions sont réalisés dans des conditions différentes.

Un leadership porteur de sens: Découvrez comment les entreprises placent la durabilité au cœur de leur stratégie.
Lire le témoignage
« Les constructeurs font probablement leurs tests dans des conditions qui n’ont rien à voir avec une exploitation réelle, en particulier sur les derniers kilomètres parcourus », avance M. Martínez.
Il suffit de comparer les performances d’un camion qui se déplace sur autoroute, à une vitesse moyenne comprise entre 65 et 80 km/h, et qui fait trois arrêts de livraison, avec celles du même camion qui parcourt une zone très vallonnée à 8 ou 16 km/h et qui effectue vingt arrêts de livraison. Les chiffres seront très différents, et dépendront du modèle. Les camions anciens ont tendance à afficher de meilleures performances que les neufs sur autoroute ; dans une étude menée avec l’entreprise de logistique mexicaine Coppel, l’équipe de M. Martínez a trouvé des exemples attestant d’une meilleure efficacité en carburant (jusqu’à 15 % de différence). L’étude a été réalisée en utilisant une vitesse, des longueurs de segments (soit la quantité d’arrêts sur un itinéraire donné) et un profil topographique moyens, et en s’appuyant sur l’apprentissage automatique pour classer les régions en fonction de ces caractéristiques – certaines étant plus résidentielles, d’autres urbaines, d’autres mixtes.
Imaginez que vous puissiez dire à un client : « Si vous êtes prêt à atteindre deux, trois, quatre jours de plus, vous pourrez réduire l’empreinte carbone de tel pourcentage. »
Josué Velázquez Martínez, Centre de transport et de logistique du MIT
Sur la base de l’analyse effectuée, l’équipe de Martínez a modélisé une réaffectation des véhicules permettant d’exploiter chacun sur les zones dans lesquelles il réalise les meilleures performances. Les résultats indiquent une réduction de 3 % du carburant utilisé – un chiffre non négligeable pour les entreprises, qui consacrent chaque année des millions à ce poste. À partir de la même hypothèse, l’équipe a également mis en place un projet pilote en conditions réelles, avec 10 véhicules sur un mois. Elle a constaté 8 % d’économies de carburant.
« Il n’est pas vraiment nécessaire d’investir, seulement de réaffecter le matériel existant », conclut M. Martínez. « Mais si vous comptez renouveler votre parc de véhicules, alors vous devez vous assurer que les nouveaux seront utilisés dans les régions attendues, puis réaffecter ceux que vous allez conserver dans votre exploitation. »
Ces résultats montrent comment la combinaison des données, des capacités de calcul et des algorithmes peut participer à améliorer le transport. La topographie a aussi son importance, ajoute M. Martínez, mais elle n’est généralement pas prise en compte dans les évaluations environnementales, car on opte plutôt pour les itinéraires les plus courts et les plus rapides, et parce que c’est un facteur complexe. Pourtant, c’est logique : monter une pente ne nécessite pas la même quantité d’énergie que de la descendre.
« Gérer ces données en utilisant le suivi GPS pour mieux cerner les faits et prendre des décisions mieux informées représente une opportunité considérable », explique-t-il. « C’est ce que j’appelle faire une petite place aux problématiques de la Supply Chain pour réduire réellement les émissions autant que possible, tout en continuant de répondre aux attentes de vos clients et d’atteindre vos objectifs commerciaux. »

Lettre d’information SAP Insights: Obtenez des insights stratégiques en vous abonnant à notre lettre d’information.
S’abonner
Une livraison le jour même est une opération complexe, car elle implique de nombreux déplacements dans les mêmes zones, avec des camions qui ne sont pas toujours pleins – ce qui augmente les émissions. Le laboratoire de recherche sur la durabilité des Supply Chains a réalisé une expérience pour voir si les consommateurs font des choix différents en fonction de leur sensibilité écologique et de leur capacité à attendre.
Dans cette expérience du « bouton vert », 52 % des clients ont effectivement changé leurs choix de livraison pour limiter leur impact environnemental. Le laboratoire a essayé différentes manières de quantifier ce paramètre pour l’utilisateur, par exemple la réduction des émissions de CO2 ou le nombre d’arbres sauvés. Avec l’exemple des arbres, les chercheurs ont établi que les clients étaient prêts à attendre quatre jours de plus en moyenne pour être livrés. Pour être précis, 70 % ont seulement déclaré qu’ils feraient ce choix, tandis que 52 % l’ont réellement fait – ce qui représente déjà plus de la moitié de la population considérée.
« Il est possible de communiquer avec transparence autour de la Supply Chain avec les clients, afin qu’ils puissent utiliser ces informations au moment d’acheter », insiste M. Martínez. Bien sûr, ce n’est pas simple. Il faut pouvoir indiquer les émissions de CO2 correspondant à chaque commande qui s’affiche dans le panier d’un consommateur, afin que ce dernier dispose de données précises et comprenne l’impact de sa décision. Cela nécessite de nombreuses analyses, voire la validation d’un tiers pour légitimer l’information fournie. Mais ce procédé représenterait un avantage significatif pour asseoir la crédibilité environnementale des marques.
« Imaginez que vous puissiez dire à un client : si vous êtes prêt à atteindre deux, trois, quatre jours de plus, vous pourrez réduire l’empreinte carbone de tel pourcentage ; vous participerez à préserver l’environnement », explique M. Martínez.
La valeur ajoutée des entrepôts locaux en termes de durabilité
Ancrer les Supply Chains localement, en alternant entre les sources d’approvisionnement et sites de production locaux et éloignés, est une autre manière de renforcer leur durabilité. Dans cette perspective, les entrepôts jouent un rôle important : construire des hangars de petite taille, à proximité de la demande, permet en effet de réduire les temps de trajet et les émissions. Les entrepôts constituent aujourd’hui le type de bâtiment commercial le plus volumineux aux États-Unis et, à en croire l’Agence américaine d’information sur l’énergie, les chiffres ne font qu’augmenter.
Il serait possible d’imposer aux entrepôts qu’ils soient autonomes énergétiquement et de les concevoir neutres en carbone. La production d’énergie alternative – par exemple, à l’aide de panneaux solaires installés sur le toit – semble couler de source et, dans certaines zones, elle est d’ores et déjà obligatoire ou subventionnée. L’Allemagne, par exemple, propose un soutien financier à l’installation d’infrastructures solaires. Aux États-Unis, il existe une multitudes d’aides fédérales et étatiques.
À Elizabeth, dans le New Jersey, East Coast Warehouse dispose de panneaux solaires sur son entrepôt, l’un des plus grands du Nord-Est des États-Unis. Kevin Daly, directeur commercial, indique que l’entreprise a récemment installé 4 900 modules solaires à haut rendement supplémentaires, qui ont généré plus de 2,5 GW en 2020. « Nos panneaux solaires ont vraiment dépassé nos attentes », se félicite-t-il.
L’entreprise s’étend dans le Maryland et en Géorgie, et envisage d’utiliser l’énergie solaire sur les entrepôts qu’elle y crée. M. Daly fait remarquer que le processus est plus simple pour les sociétés qui sont propriétaires de leurs bâtiments, entre autres parce que la communauté des développeurs s’inquiète des obligations que les panneaux peuvent engendrer, et du poids qu’ils peuvent ajouter à une structure. Ce point mis à part, ajoute-t-il, c’est une décision d’investissement, qui est souvent liée aux subventions.
À l’intérieur des entrepôts, placer les unités de gestion de stock les plus importantes et qui transitent le plus dans des emplacements rapidement et aisément accessibles est un moyen de limiter l’énergie utilisée par les chariots élévateurs.
M.Daly pense que davantage d’entrepôts adopteront l’énergie solaire et d’autres formes d’énergie alternatives. « Je ne doute pas qu’à mesure que la technologie se perfectionnera, elle deviendra encore plus efficace et plus bénéfique pour tout le monde. »

Des systèmes de gestion des entrepôts plus intelligents: Les entreprises utilisent des technologies intelligentes pour optimiser leurs opérations quotidiennes dans les entrepôts.
En savoir plus
Le fonctionnement interne des entrepôts et les livraisons sont également devenus plus durables. East Coast Warehouse a choisi de gérer ses opérations de répartition sur des tablettes et des terminaux mobiles, et de se débarrasser autant que possible du papier – et ce, dans un secteur qui en a toujours utilisé beaucoup.
Grâce aux options numériques, les chauffeurs routiers peuvent rester dans leurs camions pour gérer toutes les formalités depuis leur tablette, y compris les lettres de voiture, qui peuvent être envoyées directement aux destinataires par voie électronique. En plus de limiter la consommation de papier, ce mode de fonctionnement permet davantage de transparence. La preuve de livraison – où, quand et qui a signé – peut être intégrée à un système de gestion du transport et archivée automatiquement.
« Ce processus, qui nécessitait beaucoup d’heures de travail et d’opérations manuelles pour numériser et remplir les papiers, est devenu beaucoup plus gérable si on fait les choses de la bonne manière » conclut M. Daly.
De plus en plus d’entreprises cherchent à optimiser les opérations au sein de leurs entrepôts, comme l’explique Mme Gowda, de Chainalytics. Revenir aux principes fondamentaux de l’organisation et, par exemple, placer les unités de gestion de stock les plus importantes et qui transitent le plus dans des emplacements rapidement et aisément accessibles, est un moyen de limiter l’énergie utilisée par les chariots élévateurs.
« Le simple fait de rationaliser tout cela peut vraiment changer la donne, même au niveau de l’émission de gaz à effet de serre, car cela revient à optimiser vos opérations en tentant de tirer le meilleur parti des ressources disponibles sur le site », insiste Mme Gowda.
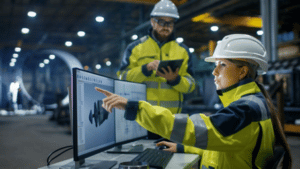
La logistique durable s’appuie sur des données
On en revient toujours à la même question : « Vos données sont-elles de bonne qualité ? » Parce qu’il ne suffit plus d’utiliser des données d’historique ou d’estimer des paramètres tels que les émissions générées par les camions.
Désormais, des sociétés tierces proposent les outils nécessaires pour déterminer les émissions de CO2 réelles d’un véhicule donné, à partir des informations opérationnelles collectées. Ces outils sont capables de transmettre ces informations sur des tableaux de bord, de manière à faciliter une approche stratégique dans la réflexion et les actions à mener – et à créer ainsi une Supply Chain plus cohérente de bout en bout.
Autre grand objectif d’un grand nombre de marques et de fabricants : pouvoir ventiler les émissions par produit, de leur conception à leur mise hors service. Ces informations, réclamées par les consommateurs, peuvent constituer un facteur de différenciation considérables pour une marque si elles sont bien compilées et communiquées avec précision (c’est-à-dire sans déclarations non étayées ni « greenwashing »).
D’après Mme Gowda, on prête de plus en plus d’attention à la logistique inverse, car les entreprises modernes consacrent davantage d’analyses à mieux comprendre le coût environnemental de leurs produits, tout au long de leur cycle de vie. Les clients de Chainalytics souhaitent aujourd’hui élaborer des scénarios qui accordent la priorité aux gaz à effet de serre, et non aux coûts. Dans ce domaine, la création d’un jumeau numérique se révèle très utile.
« Il devient un peu difficile de répondre à ce genre de questions sans disposer d’une plateforme de jumeau numérique complètement fonctionnelle », explique Mme Gowda. « Mais nous sommes définitivement plus efficaces qu’il y a dix ans. Nous sommes vraiment en mesure de comprendre notre propre impact, et nous essayons de créer davantage de solutions pour favoriser des Supply Chains plus vertes. »
Nous sommes vraiment en mesure de comprendre notre propre impact, et nous essayons de créer davantage de solutions pour favoriser des Supply Chains plus vertes.
Shimon Gowda, responsable de conception de la Supply Chain chez Chainalytics
Rationaliser l’approvisionnement et les prévisions de la demande peut également renforcer la durabilité, indique Mme Gowda. Tout au long de la Supply Chain, chaque acteur dispose de ses propres métriques prévisionnelles. Mais s’il y a une faille en amont, elle se répète et s’amplifie en aval. Cela peut entraîner une surproduction de la part de toutes les entreprises de la chaîne, ce qui signifie des déchets ou des produits excédentaires qui se retrouvent dans les entrepôts après avoir voyagé dans le monde entier. « C’est typiquement le scénario que nous cherchons à éviter quand nous essayons d’évoluer vers un fonctionnement plus durable, neutre en carbone », conclut-elle.
Un concept de Supply Chain appelé « planification collaborative et réapprovisionnement prévisionnel » est en train de gagner du terrain. C’est un autre exemple de scénario dans lequel les jumeaux numériques jouent un rôle essentiel, car chaque acteur de la Supply Chain peut voir les données transférées par les autres. Par exemple, le fabricant est en mesure de consulter les prévisions du détaillant final trois mois à l’avance. Tout problème peut ainsi être identifié et résolu plus tôt. « Cette solution renferme un vrai potentiel de progression vers l’économie circulaire, car elle implique de se montrer minimaliste dans sa consommation, dans ses transferts… sur tous les points, en somme », explique Mme Gowda.
À mesure que les entreprises s’attachent à évaluer l’impact environnemental réel de leur Supply Chain et de leurs opérations logistiques, les livraisons se rationalisent, de manière à générer moins de déchets et moins d’émissions. Un bonne nouvelle pour l’environnement comme pour les clients !
Note des éditeurs : Découvrez des idées logistiques plus durables et des exemples pratiques dans L’économie circulaire se popularise ; 5 vérités sur la Supply Chain dans le monde post-COVID-19 ; et Sur la terre, la mer et dans les airs : des technologies émergentes pour maîtriser le changement climatique.
The post La logistique durable en mouvement Till Dengel, Richard Howells | 14 min de lecture appeared first on SAP France News.
Source de l’article sur sap.com